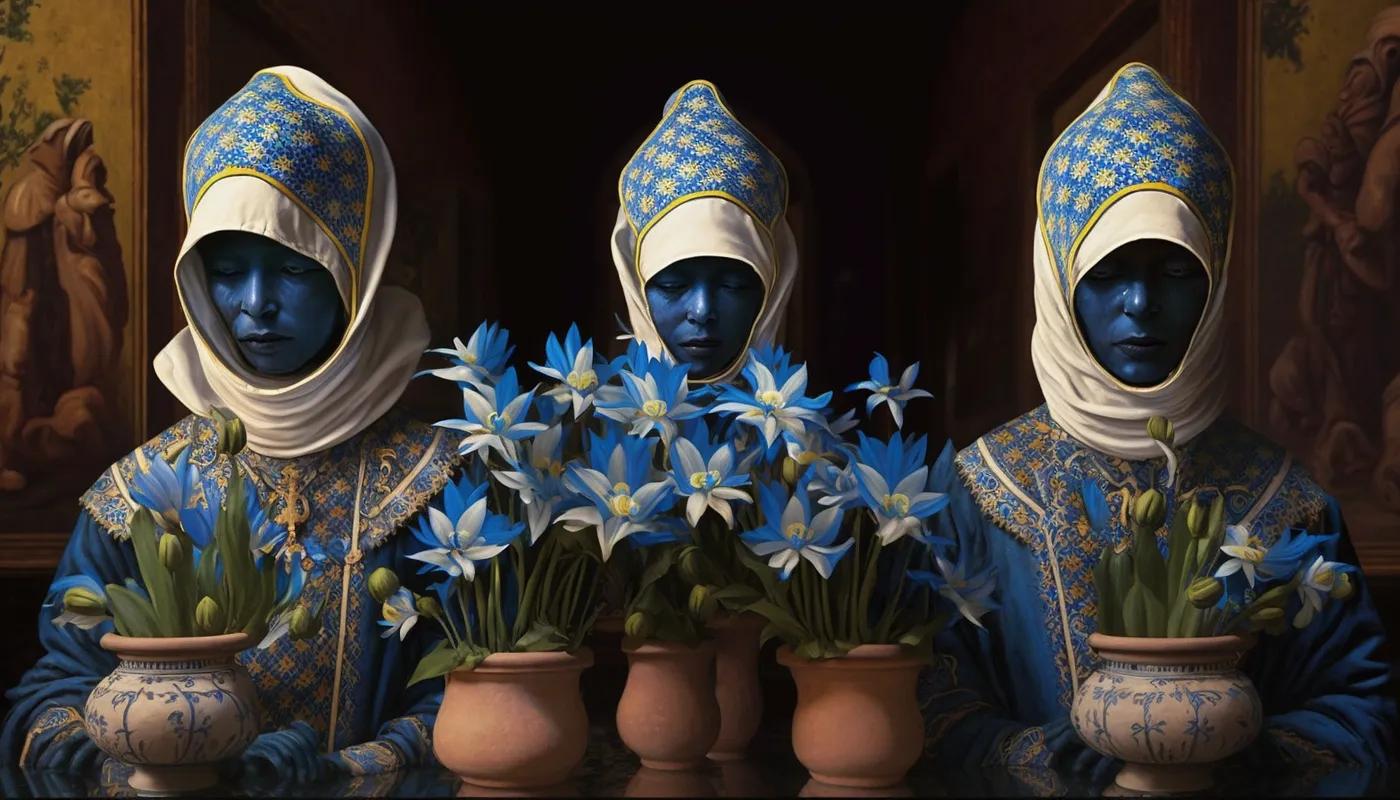
It’s early March and the glimpses of spring are in the air. A new cycle begins. If you have been around the last three months, you couldn’t have avoided seeing some cryptic names, such as ChatGPT, and the resurrection of a once-archaic term: Artificial Intelligence (AI). For those who remember, before we preferred a more technical, and narrower term — machine learning or deep learning.
ChatGPT belongs to a family of Large Language Models (LLMs), which are capable of generating or transforming text. To simplify the terminology, in the rest of the article we will often use the name ChatGPT as a placeholder for any advanced LLM. Examples of capabilities include generating a poem in the style of any writer you can think of, explaining a complex function or program, or interacting in a meaningful and enriching way as a chat bot — all of this in plain English or any other supported language. Generative AI is then an umbrella term for ChatGPT-like systems applied to different modalities besides text, such as images and music.
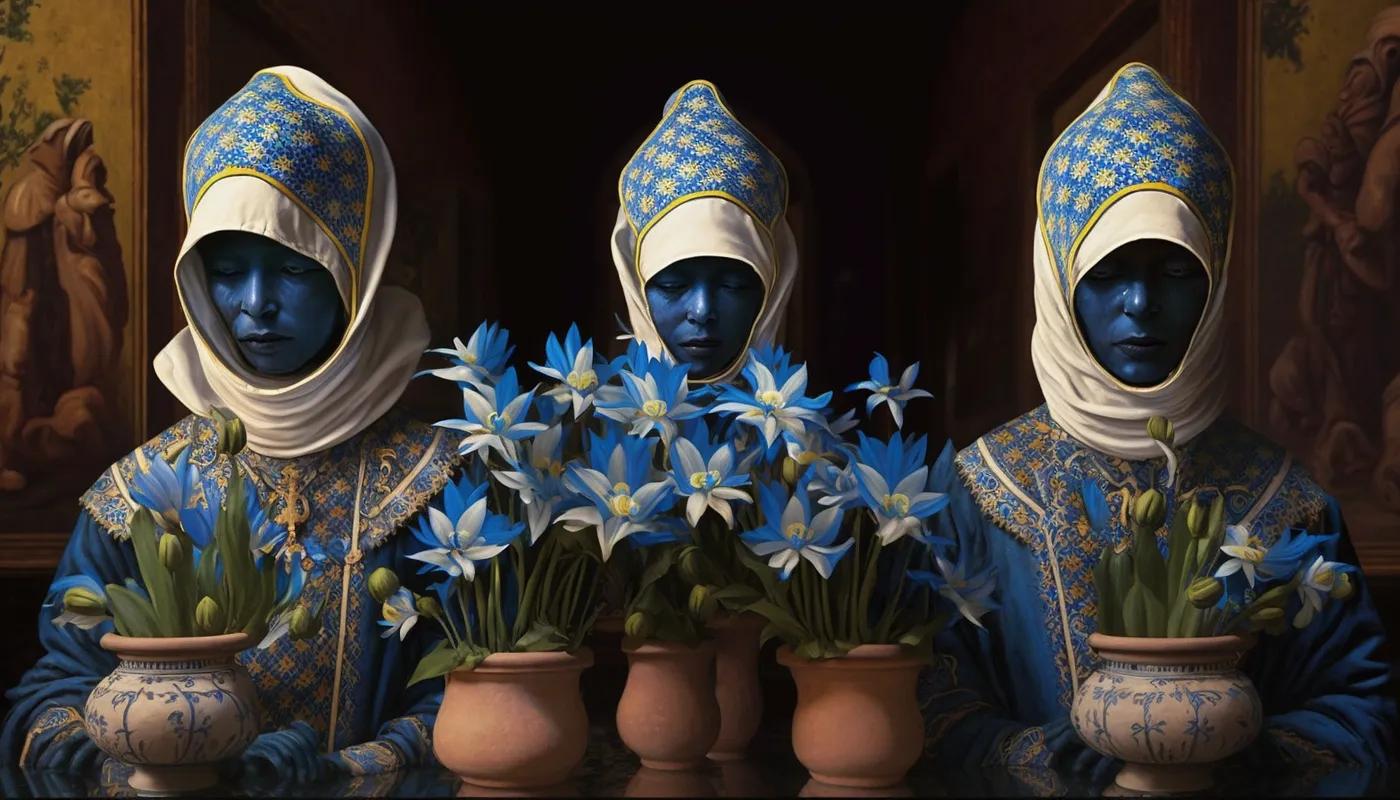
Compared to its predecessor, although there have been several shortcomings reported, ChatGPT stands out and even shocks people due to the depth of answers and logical reasoning, which was never explicitly put or hardwired into the system. The magnitude of its societal implications will likely overshadow even the most recent and impactful technological leap — the origin of the smartphone. Yes, we have had machine learning for a few decades, but this time we feel that, by following the trajectory paved by ChatGPT, Artificial General Intelligence (AGI) is within reach; predicted to occur by 2040 (the current community prediction).
I cannot help myself but when events of this magnitude happen, everything looks strangely familiar. Our pop culture is full of black swan events, technological singularities, and pandemics. We have speculated about AI many times before and we know the flood is coming; it touches every aspect of our lives, including the economy, education, ethics, and philosophy.
Main Improvements
The magical piece responsible for this technological advancement is the transformer. Without going too deep, let’s state that a transformer, originally introduced by Google researchers to tackle language translation, is a type of neural network that takes one sentence and outputs a different sentence (in the same or a different language). By feeding it with the entire Wikipedia, books, and the entirety of the scrapable internet, it can create a rich representation of each word while taking contextual information into account.
The model of transformer has been around for more than five years, so the question is why now? There are several factors at play, but the largest performance and accuracy gain is due to the training method called Reinforcement Learning from Human Feedback (RLHF). In essence, a human trainer provides feedback (or reward) on the generated text to guide the system and its trajectory to be in line with human expectations — tone, phrasing, values, and emotional charge of the text. If absent, the output is often too dry and much less engaging. In other words, the goal of essentially pleasing us is more important than factual correctness and ultimately the truth, which will be discussed later. It has been shown that the system trained by human feedback is better (more human-aligned) than even a system 100 times larger.
The second important and slightly unexpected innovation is logical inference (although one can argue it is still fairly basic). The most likely explanation is that the system acquired this ability by being exposed to an immense amount of code. Specifically, it learned to infer logical effects by abstracting the procedural flow of commands. Note that it was something completely unintentional, a genuine emergent property of the system.
The last contributing factor is the size. In the field of machine learning, there is a growing number of proponents of so-called scalability hypothesis claiming that we already have good enough neural nets, in terms of their structure and architecture. The only thing remaining is to enlarge and feed them with more data. For illustration, GPT-2 had around 1.5 billion parameters, but its successor GPT-3 has 100 times more. Even if this assumption holds, the realization might face serious practicality challenges going forward. In particular, the costs and computing power required for training such huge models increases hyper-exponentially (Figure bellow), which is unsustainable from the hardware perspective. In any case, the beneficiaries of the current AI boom are chip manufactures, such as NVIDIA, Intel, and AMD.
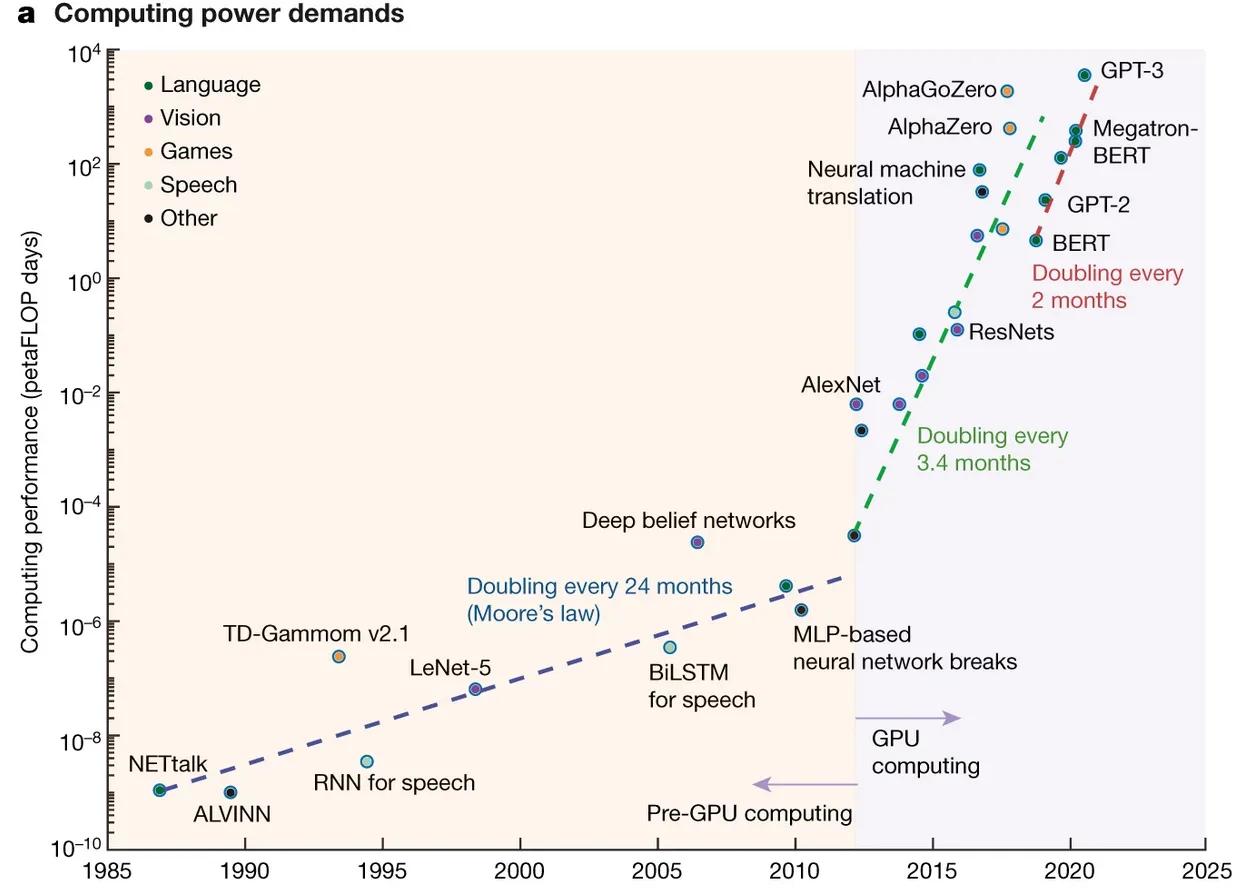
Socio-economics
Contrary to what was predicted 10 years ago, the current wave of AI targets and threatens mostly white-collar jobs, such as graphic designers, programmers, copywriters, journalists, and lawyers. The dominant narrative back then was that AI would make blue-collar jobs obsolete, first in factories, then moving up to truckers and taxi drivers due to autonomous cars, post office workers, etc. We now know that this vision failed and only limited use cases have come to fruition. Several factors are at play, but the main show-stopper is that to replace a worker in a factory with a single-purpose robot that costs 10k is simply ineffectual. Additionally, the physical world is messy, open, and unpredictable, which imposes difficult requirements on dexterity and robustness. On the other hand, generative AI deals purely with information flows, such as text and images. Training such a model is expensive and time-consuming, but once it is done, it can be scaled to millions of tasks and users with operational costs in a sub-cent range per call.
The jobs won’t perish overnight, but the productivity race is on. As Sam Altman, CEO of OpenAI, the company behind ChatGPT stated: “The marginal cost of intelligence and energy will rapidly go towards zero.” To stay competitive, we must embrace this technology in the form of copilots and assistants. Dozens of companies emerge every week with generative AI at the very core of their technological stacks, disrupting the status quo in many industries. One such company is Cequence, where I work as CSO. We keep a tight loop on what’s happening and closely monitor AI trends with the approach characterized by fast prototyping and integration applied to contract management. My previous post about our Scala client for OpenAI API can be found here.
Early AI, called cybernetics, which came to prominence in the 1950s, clearly overpromised in the sense of early futurists and Jules Verne’s romanticism. Nevertheless, the focus on feedback loops and learning based on the model of artificial neuron set the stage for what came next. Now thanks to the computing power as well as novel neural network components, such as transformer, we are definitely in the AI summer. Because of the flipping of the perspective we shouldn’t however do the opposite mistake of underestimating AI’s capabilities and implications. People in general do a very poor job imagining an exponential growth.
The Internet as it has existed over the last 20 years is an intricate ecosystem of websites, with a hierarchy determined by Google rank and fueled by advertising revenue. Currently, with the “ask me anything” approach, the system synthesizes and presents a final answer to your query, rather than providing a list of websites with fractional information. This means the classic search-and-browse model, and everything around it, will likely cease to exist. Whoever is cheering the development should be reminded that, since we do not need to step out of the system, the consequence is more centralization of not just revenue, but also control and trust. That’s why it is of strategic importance that more companies step in and provide products comparable to OpenAI, which has been happening to some extent (Google’s Bard, Meta’s LLaMA). From my perspective, we also need to ensure that decentralized, open-source initiatives spring up to counterbalance the big tech and provide fully transparent intelligence essentially as a public resource. More dystopian suggestion is to embrace an inevitable netocrat-consumtariat society, where a handful of AI companies control the market and, as a result, are heavily taxed, which is then distributed to citizens as universal basic income to offset job loss. Interestingly, Sam Altman is also behind Worldcoin, a crypto project that aims to provide global digital identities by scanning people’s irises.
Ethics and Moral
Disruptive tech has long been at odds with governments and societies, especially when it comes to moral and public good. Our relationship with technology is schizophrenic: on one hand, we welcome anything that helps us to save time and money; on the other, we cheer and even laugh when technology fails. We consider it as a proof that we still run the show and of our cognitive and creative superiority. This perception asymmetry causes a single malfunction (e.g., a crash of a self-driving car) to blow out of proportion.
In the field of generative AI, the stakes are high, so we can expect lawsuits and strong opposition — some justified (e.g., artistic work used for training without consent), others purely defensive (e.g., industries that are no longer required). The technology moves so fast that any attempt to regulate it at a finer level is destined to be outdated the moment it comes into effect, especially aspects requiring any kind of societal consensus.
In the EU, generative AI is already the third recent technological wave the EU is set to miss out on (after machine learning and crypto). Rather than promoting businesses to innovate and embracing the technology, the EU is yet again following its old playbook to become the regulatory leader — a title that one assigns to themselves, if everything else is taken.
We should of course be careful. Governments must require platforms, regardless of the domain, to comply with strict privacy and security standards, and we should stop accepting that our data is exploited and sold. If an AI system makes an autonomous action, then safety and accountability need to be addressed as well. On the other hand, trying to define universal content moderation policies or a set of biases that the system must avoid during its training phase is practically unachievable. Rather than focusing on and defining the truth, which is increasingly elusive, it is more productive if governments support decentralized, public, AI-based alternatives with a different set of incentives, where citizens can easily opt-in and out as they would in a network state.
From Descriptive to Generative to Prescriptive
One of the unintended consequences of training ChatGPT on an enormous dataset is that it understands instructions and contextual descriptions such as “write me a song about cats playing didgeridoo on Mars.” Researchers at OpenAI found that a set of filters preventing biases can be actually defined in a similar manner. Traditionally, if we want a training dataset not to contain a certain bias (e.g., racism), a data curator would need to meticulously cross out anything that could potentially be biased. Besides costs, poor scalability, and questionable validation metrics, a resulting “safe” dataset is miniscule compared to what’s out there, negatively affecting the overall performance and accuracy of the system. Since ChatGPT understands the context of, for example, racism, an alternative bias-removal method is to simply specify “don’t be racist” at the beginning of each prompt.
Because filters like that can be explicitly and easily stated as a part of an invisible set of presumptions for each conversation, other rules such as “be informative, visual, logical, and actionable”, as well as “positive, interesting, entertaining, and engaging” (actual filters of ChatGPT model in Bing) then define what kind of answers we obtain from the system, and what is effectively censored or sugarcoated.
For instance, it was reported that ChatGPT was able to generate a poem honoring Biden, but refused to make one that praised Trump. As you can expect, it didn’t take long and a political mirror image of ChatGPT — called RightWingGPT — emerged. This division shows that various political or (sub)cultural groups might desire their own generative models representing their values, biases, and beliefs, with filters that are transparent to each community, similar to moderation rules.
Funnily enough, the generative AI landed precisely at the point where the failed experiment of social networks ended. Consequently, the center has been deserted, and we slowly accept that achieving a neutral truth layer was too naive and the fragmentation into Reddit-like groups that followed is beyond repair.
The decay of truth began, however, some time ago and was brought to prominence by postmodernists in the intellectual circles of the 1970s and 1980s; centered around the idea that everything is a social construct and the rest is a power/control play. Philosophical postmodernism is relevant to understanding the current situation and what comes next, due to the existence of models. In particular, we have moved from descriptive models, where observations of a target modality (e.g., text, music, and image) are generalized to serve validation and prediction, to generative models, where, as Baudrillard nicely put it, the models become radiant.
Due to the filters and training by human feedback, the text generated by ChatGPT is aligned with our values and thematic expectations, but not necessarily with hard facts. There are many examples when ChatGPT has “hallucinated” something (the term used by others) that wasn’t true. However, due to the generalization employed, the answer is often probable — for example, a certain bar matching a given description may exist, but in a different town. The generation of the “hyper-real” — the double without an origin, without an anchor to the “real” world — is nothing new. Through stories, ideologies, culture, and religion, we have been building a new virtual level, a simulacrum, for ages. With generative AI, this evolution is being given a steroid shot, spinning combinatorial orbits of the possible as well purely synthetic. We can, of course, employ generative AI for the least speculative approach — to create fictional books, music, video, or anything else for entertainment purposes (perhaps, later, in some kind of metaverse) but we know it won’t stop there.
At the granularity of individuals, the collective referentials are further destroyed regardless of one’s initial beliefs. Each person can have their own generative AI, a “double” that produces custom and personalized news feeds, movies, and music for internal consumption, and another for external use — effectively representing them to the outside world. For example, I didn’t want this article to be generic and just informative, but to represent me, my beliefs, and ideas. When it comes to generative AI, we want it to reply to emails exactly as we would — or, let’s admit it, maybe with a slight facelift — but still fully aligned with who we are.
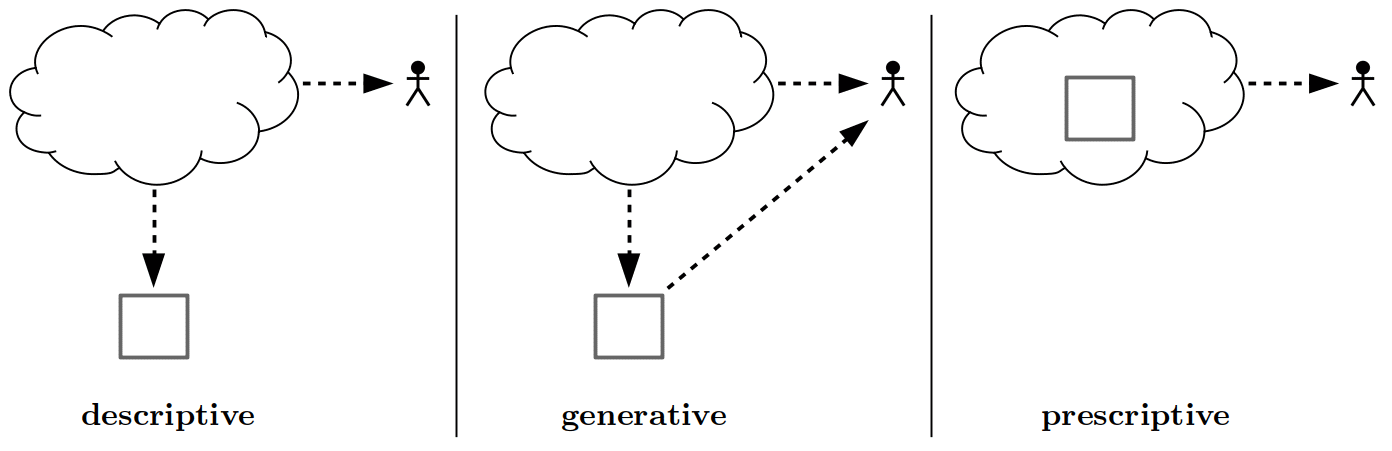
At the final stage, we transitioned from generative to prescriptive, where the hard truth and the harsh reality was completely abandoned (Baudrillard: “desert of the real”), and the actionable world became a pure simulacrum. The panic production of goods was replaced by a panic production of the hyper-real (narration factory); consumed in isolation by individuals in synthetic echo chambers designed to please us.
As J.L. Austin argued, the purpose of language is not simply about making true or false statements, i.e. referring and predicating, but rather it is an act and therefore its purpose is primarily performative. This is also true for language models and generative AI — instead of focusing on truth, we turn our attention to action, with the goal of triggering a change brought about by the strong intentionality of the narrator.
Outro
The generative AI, as a technological and societal revolution, will enable new industries and productivity levels never seen before. It is up to governments and all of us to mitigate centralization risks and guarantee transparent execution and customization of models based on someone’s preference.
The paradigm has shifted and it is going to change everything, just like in the movie “Everything, Everywhere, All at Once” — a nice metaphor of what we are experiencing: supercharged radiant models producing combinatorial, intertwined orbits of future meanings. All of this crowned by the transition from generation (the latest stage of consumer capitalism) to the actual prescription of reality. The war has been lost, and we should stop obsessing over the hard, collective truth — which is a funny thing to hear from a scientist, I guess. The main, and probably only non-nihilistic, response to the postmodern world of generative AI is to act; to go out there, build, and create things. The future might be bright, after all, if we play it right.
Hope you enjoyed the article; appreciate any clap/follow!
Acknowledgement: The post has been reviewed by Jens Bürger from Leuven.AI (thanx!), and proof-read by text-davinci-003
model from OpenAI (mostly articles, yay) - opted out of the data collection. Last but not least, I am grateful for the support from Cequence!